Cédric Chevalier travaille dans une équipe de R&D du CEA la spécialité est le calcul parallèle pour la simulation numérique à grande échelle.
Ses principaux centres d’intérêts sont le calcul combinatoire, en particulier les problèmes de partitionnement, de maillage, de graphe ou de nombres ; et les algorithmes d’algèbre linéaire creuse.
Principaux thèmes de recheche
Partitionnement
Pour les problèmes de répartition de charge ou de numérotation. Ces problèmes combinatoires sont en général traités sous la forme de problèmes de partitionnement de nombres, de vecteurs de nombres, de graphes ou de maillages.
Deux thèses ont été conduites sur ce thème et une troisième est en cours. Le logiciel Coupe est le fruit de ses travaux.
Algèbre linéaire
Plus précisément l’algèbre creuse et les méthodes itératives pour la résolution de grands systèmes linéaires. Les travaux portent avant tout sur l’implantation et la composition des logiciels de résolution, dans le cadre du logiciel Alien.
Performance et précision numérique
Les optimisations logicielles et matérielles autour des calculs flottants peuvent changer les résultats. L’étude de ces changements et comment les gérer a donné lieu à une thèse, dont est issu le logiciel Shaman.
Autres centres d’intérêts
Calcul haute performance
La bonne exploitation des ressources des calculateurs est cruciale. Par l’étude de mini-applications, il est possible de comprendre comment améliorer l’utilisation des nouvelles architectures. Cette activité donne lieu à de nombreux stages.
Techniques de programmation
De nouveaux langages et paradigmes de programmation voient le jour, promettant des codes plus robustes et plus performants. Leur évaluation dans un contexte de calcul scientifique est important et donne lieu à des stages. En particulier l’écosystème Rust fait l’objet d’un suivi approfondi.
SIAM International Meshing Roundtable 2023, Springer Nature Switzerland, p. 43-63, 2023
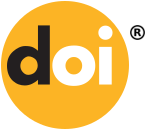
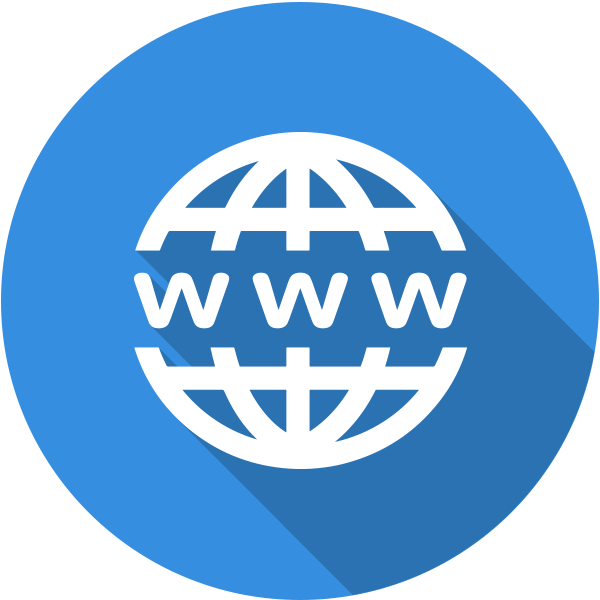
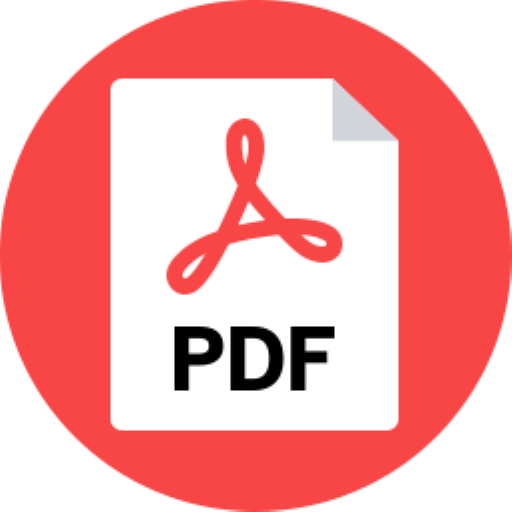
abstract
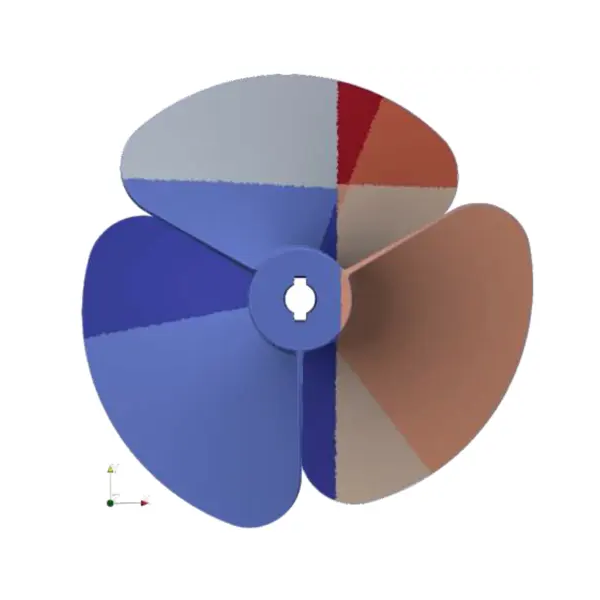
Abstract
This paper presents Coupe, a mesh partitioning platform. It provides solutions to solve different variants of the mesh partitioning problem, mainly in the context of load-balancing parallel mesh-based applications. From partitioning weights ensuring balance to topological partitioning that minimizes communication metrics through geometric methods, Coupe offers a large panel of algorithms to fit user-specific problems. Coupe exploits shared memory parallelism, is written in Rust, and consists of an open-source library and command line tools. Experimenting with different algorithms and parameters is easy. The code is available on Github.
Euro-Par 2022 International Workshops, Glasgow, UK, August 22–26, 2022, Revised Selected Papers, Glasgow, United Kingdom, 2023
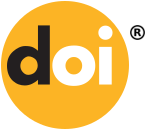
abstract
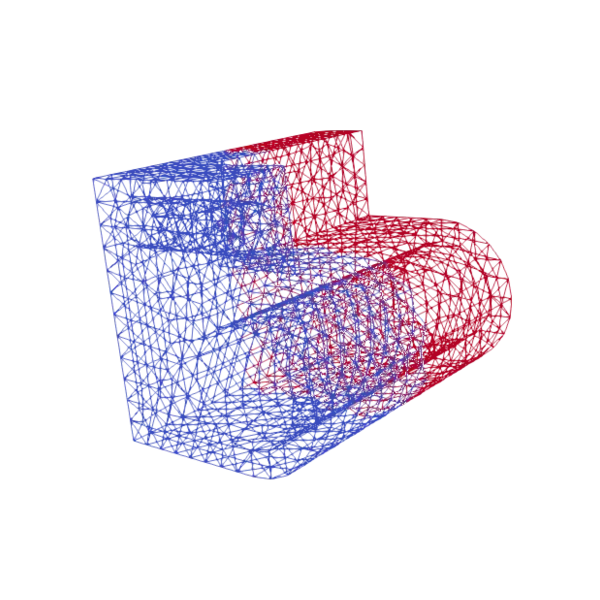
Abstract
Mesh partitioning used for load balancing in distributed numerical simulations is typically managed with tools that are good enough but not optimal. Their use scope is not explicitly dedicated to load balancing, and they cannot make use of all available information. In this paper, the mesh partitioning problem and the context for its use are precisely defined. Then, existing tools are presented, along with their characteristics and features that are missing. Finally, a new partitioning platform – the subject of my PhD thesis – is presented: its architecture, software engineering choices made along the way, and how it can be the best fit for load balancing distributed simulations. The platform is open-source and is hosted on GitHub: https://github.com/LIHPC-Computational-Geometry/coupe .
ACM Transactions on Mathematical Software, Volume 48, Issue 4, 2023
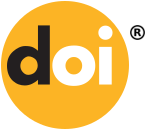
abstract
Abstract
Floating-point numbers represent only a subset of real numbers. As such, floating-point arithmetic introduces approximations that can compound and have a significant impact on numerical simulations. We introduce encapsulated error, a new way to estimate the numerical error of an application and provide a reference implementation, the Shaman library. Our method uses dedicated arithmetic over a type that encapsulates both the result the user would have had with the original computation and an approximation of its numerical error. We thus can measure the number of significant digits of any result or intermediate result in a simulation. We show that this approach, although simple, gives results competitive with state-of-the-art methods. It has a smaller overhead, and it is compatible with parallelism, making it suitable for the study of large-scale applications.
2020 Proceedings of the SIAM Workshop on Combinatorial Scientific Computing, p. 85-95, 2020
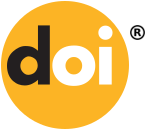
abstract
Abstract
Running numerical simulations on HPC architectures requires distributing data to be processed over the various available processing units. This task is usually done by partitioning tools, whose primary goal is to balance the workload while minimizing inter-process communication. However, they do not take the memory load and memory capacity of the processing units into account. As this can lead to memory overflow, we propose a new approach to address mesh partitioning by including ghost cells in the memory usage and by considering memory capacity as a strong constraint to abide. We model the problem using a bipartite graph and present a new greedy algorithm that aims at producing a partition according to the memory capacity. This algorithm focuses on memory consumption, and we use it in a multi-level approach to improving the quality of the returned solutions during the refinement phase. The experimental results obtained from our benchmarks show that our approach can yield solutions respecting memory constraints for instances where traditional partitioning tools fail.